Qitian Zeng, Ph.D. Student
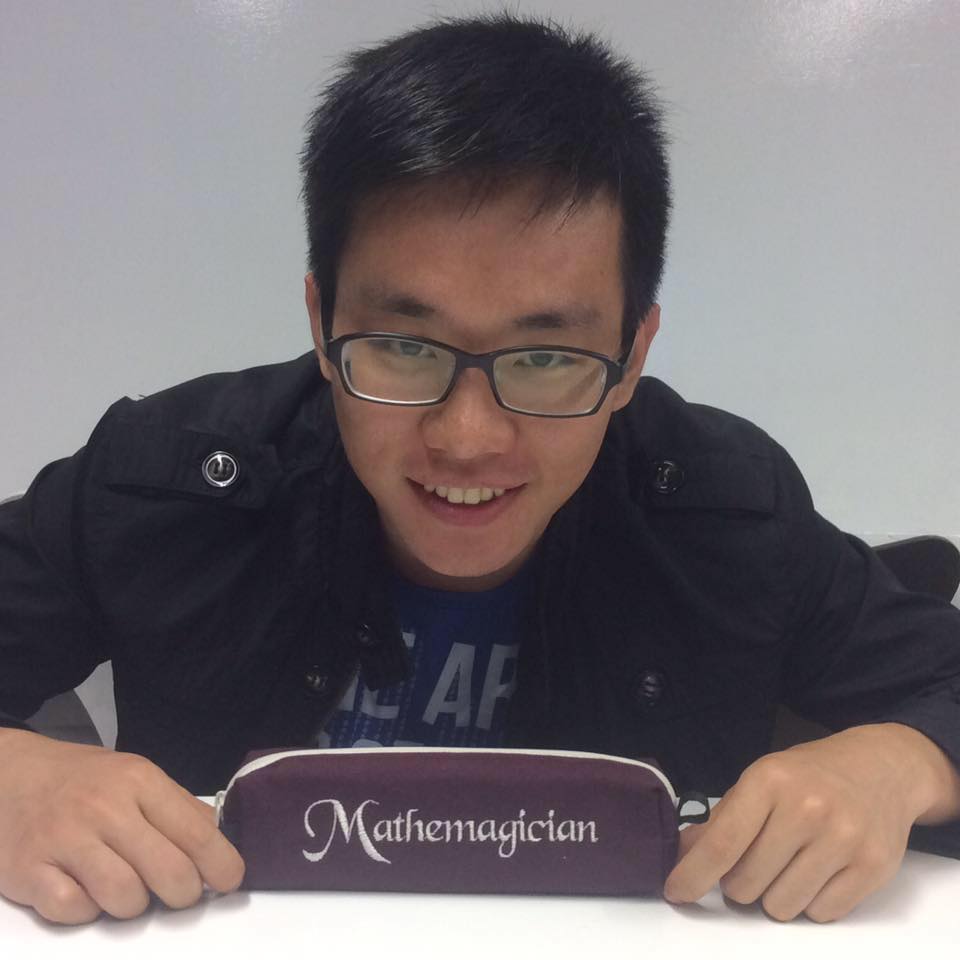
I received my Bachelor in Economics with minor in Math in 2014 from Nankai University in China and Master of Mathematical Finance in 2016 at Illinois Tech. I started PhD in Computer Science in 2017 at Illinois Tech.
Awards
- UPE Honorable Mention Award (2018)
Teaching
I have been TA for the following courses:- 2017 Fall: CS425 - Database Organization
- 2018 Fall: CS425 - Database Organization
Research Projects
I am involved in the following research projects:- -
- HCDF - A Hybrid OS / Runtime for Task-based In-memory Query Processing
Collaborators
I am collaborating with:- Kyle Hale - Illinois Institute of Technology
Publications
-
Putting Things into Context: Rich Explanations for Query Answers using Join Graphs
Chenjie Li, Zhengjie Miao, Qitian Zeng, Boris Glavic and Sudeepa Roy
Proceedings of the 46th International Conference on Management of Data (2021), pp. 1051–1063.@inproceedings{LM21, author = {Li, Chenjie and Miao, Zhengjie and Zeng, Qitian and Glavic, Boris and Roy, Sudeepa}, booktitle = {Proceedings of the 46th International Conference on Management of Data}, pages = {1051–1063}, projects = {Explanations beyond Provenance}, title = {Putting Things into Context: Rich Explanations for Query Answers using Join Graphs}, pdfurl = {https://dl.acm.org/doi/pdf/10.1145/3448016.3459246}, doi = {10.1145/3448016.3459246}, keywords = {Provenance; Explanations}, venueshort = {SIGMOD}, reproducibility = {https://github.com/IITDBGroup/CaJaDe}, video = {https://www.youtube.com/watch?v=puhCAnFuPR4&list=PL3xUNnH4TdbsfndCMn02BqAAgGB0z7cwq}, longversionurl = {https://arxiv.org/pdf/2103.15797}, year = {2021} }
In many data analysis applications there is a need to explain why a surprising or interesting result was produced by a query. Previous approaches to explaining results have directly or indirectly relied on data provenance, i.e., input tuples contributing to the result(s) of interest. However, some information that is relevant for explaining an answer may not be contained in the provenance. We propose a new approach for explaining query results by augmenting provenance with information from other related tables in the database. Using a suite of optimization techniques, we demonstrate experimentally using real datasets and through a user study that our approach produces meaningful results and is efficient.
-
Playing Fetch with CAT - Composing Cache Partitioning and Prefetching for Task-based Query Processing
Qitian Zeng, Kyle Hale and Boris Glavic
International Workshop on Data Management on New Hardware (2021).@inproceedings{ZHG21, author = {Zeng, Qitian and Hale, Kyle and Glavic, Boris}, title = {Playing Fetch with CAT - Composing Cache Partitioning and Prefetching for Task-based Query Processing}, keywords = {Cache; Main-memory Databases; HCDF}, booktitle = {International Workshop on Data Management on New Hardware}, projects = {HCDF}, venueshort = {DaMoN}, doi = {10.1145/3465998.3466016}, pdfurl = {http://cs.iit.edu/%7edbgroup/assets/pdfpubls/ZH21.pdf}, year = {2021}, pages = {} }
-
Going Beyond Provenance: Explaining Query Answers with Pattern-based Counterbalances
Zhengjie Miao, Qitian Zeng, Boris Glavic and Sudeepa Roy
Proceedings of the 44th International Conference on Management of Data (2019), pp. 485–502.@inproceedings{MZ19, author = {Miao, Zhengjie and Zeng, Qitian and Glavic, Boris and Roy, Sudeepa}, booktitle = {Proceedings of the 44th International Conference on Management of Data}, keywords = {Provenance; Explanations; Cape}, pages = {485--502}, pdfurl = {http://cs.iit.edu/%7edbgroup/assets/pdfpubls/MZ19.pdf}, reproducibility = {https://hub.docker.com/repository/docker/iitdbgroup/2019-sigmod-reproducibility-cape}, doi = {10.1145/3299869.3300066}, video = {https://av.tib.eu/media/42903}, projects = {Explanations beyond Provenance}, slideurl = {https://www.slideshare.net/lordPretzel/2019-sigmod-going-beyond-provenance-explaining-query-answers-with-patternbased-counterbalances}, title = {Going Beyond Provenance: Explaining Query Answers with Pattern-based Counterbalances}, venueshort = {SIGMOD}, year = {2019} }
Provenance and intervention-based techniques have been used to explain surprisingly high or low outcomes of aggregation queries. However, such techniques may miss interesting explanations emerging from data that is not in the provenance. For instance, an unusually low number of publications of a prolific researcher in a certain venue and year can be explained by an increased number of publications in another venue in the same year. We present a novel approach for explaining outliers in aggregation queries through counterbalancing. That is, explanations are outliers in the opposite direction of the outlier of interest. Outliers are defined w.r.t. patterns that hold over the data in aggregate. We present efficient methods for mining such aggregate regression patterns (ARPs), discuss how to use ARPs to generate and rank explanations, and experimentally demonstrate the efficiency and effectiveness of our approach.
-
CAPE: Explaining Outliers by Counterbalancing
Zhengjie Miao, Qitian Zeng, Chenjie Li, Boris Glavic, Oliver Kennedy and Sudeepa Roy
Proceedings of the VLDB Endowment (Demonstration Track). 12, 12 (2019) , 1806–1809.@article{MZ19a, author = {Miao, Zhengjie and Zeng, Qitian and Li, Chenjie and Glavic, Boris and Kennedy, Oliver and Roy, Sudeepa}, date-modified = {2019-08-02 09:14:13 -0500}, journal = {Proceedings of the VLDB Endowment (Demonstration Track)}, keywords = {Outliers; Intervention; Cape; Explanations}, pdfurl = {http://www.vldb.org/pvldb/vol12/p1806-miao.pdf}, projects = {Explanations beyond Provenance}, pages = {1806-1809}, volume = {12}, issue = {12}, doi = {10.14778/3352063.3352071}, title = {CAPE: Explaining Outliers by Counterbalancing}, venueshort = {{PVLDB}}, year = {2019} }
In this demonstration we showcase Cape, a system that ex- plains surprising aggregation outcomes. In contrast to previous work which relies exclusively on provenance, Cape applies a novel approach for explaining outliers in aggregation queries through counterbalancing (outliers in the opposite direction). The foundation of our approach are aggregate regression patterns (ARPs) based on which we defined outliers, and an efficient explanation generation algorithm that utilizes these patterns. In the demonstration, the audience can run aggregation queries over real world datasets, and browse the patterns and explanations returned by Cape for outliers in the result of such queries.
-
GProM - A Swiss Army Knife for Your Provenance Needs
Bahareh Arab, Su Feng, Boris Glavic, Seokki Lee, Xing Niu and Qitian Zeng
IEEE Data Engineering Bulletin. 41, 1 (2018) , 51–62.@article{AF18, author = {Arab, Bahareh and Feng, Su and Glavic, Boris and Lee, Seokki and Niu, Xing and Zeng, Qitian}, bibsource = {dblp computer science bibliography, https://dblp.org}, biburl = {https://dblp.org/rec/bib/journals/debu/ArabFGLNZ17}, journal = {{IEEE} Data Engineering Bulletin}, keywords = {GProM; Provenance; Annotations}, number = {1}, pages = {51--62}, pdfurl = {http://sites.computer.org/debull/A18mar/p51.pdf}, projects = {GProM; Reenactment}, timestamp = {Fri, 02 Mar 2018 18:50:49 +0100}, title = {{GProM} - {A} Swiss Army Knife for Your Provenance Needs}, venueshort = {Data Eng. Bull.}, volume = {41}, year = {2018}, bdsk-url-1 = {http://sites.computer.org/debull/A18mar/p51.pdf} }